MMM & Generative Marketing Attribution
Marketing Mix Modelling vs Media Mix Modelling vs Multi-touch Attribution vs Unified Marketing Measurement vs Generative Marketing Attribution - what does it all mean? How does AI fit in?
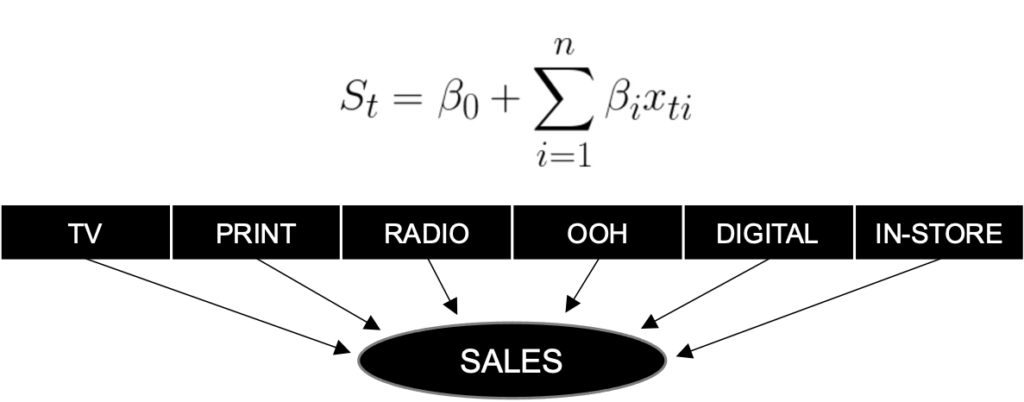
- Marketing Mix Modelling vs Media Mix Modelling
Marketing Mix Modelling (MMM) and Media Mix Modelling (also, confusingly, MMM) are indispensable methodologies in the marketing to help dissect the effectiveness of marketing efforts. However, these two terminologies, though often used interchangeably, bear distinct implications.
The primary difference between Media Mix Modelling and Marketing Mix Modelling lies in their scope of analysis.
Marketing Mix Modelling, sometimes referred to as econometric modeling, is a statistical technique used to quantify the influence of various marketing variables on sales, market share, or what marketing KPI that advertiser cares about. It looks at the entire spectrum of the marketing ecosystem, taking into account online and offline channels, seasonality, competition, market conditions, and the ‘Four Ps’ of marketing: product, price, promotion, and place.
Media Mix Modelling, on the other hand, is a subset of MMM. It narrows its focus to the ‘Promotion’ aspect of the Marketing Mix Model, scrutinising the performance of different media channels and their impact on sales or other desired outcomes. Media agencies tend to care about this flavour of MMM, whereas advertisers (and their CFOs, especially), tend to care more about the holistic MMM approach.
How does a MMM model work?
A marketing mix model takes into account marketing variables such as spend across channels, promotional activities, competitive actions, economic indicators and other non-media factors relative to the advertiser (pricing, distribution, PR etc). By identifying patterns and establishing correlations between these marketing inputs and outcomes, the MMM model provides valuable insights into the effectiveness of different marketing channels and activities. This empowers businesses to optimise future spending to deliver maximum return on investment. MMM is not a new concept, its origins trace back to the mid-20th century when scholars began to explore the impact of marketing inputs on market outcomes. However, it wasn’t until the 1980s, with the advent of personal computers and statistical software, that MMM began to take its modern form, gaining prominence in commercial marketing decisions. This was long before the intense media fragmentation that we enjoy/endure today.
Multi-Touch Attribution
In the early 2000s, the emergence of digital marketing channels gave birth to a new method for understanding marketing effectiveness called Multi-Touch Attribution (MTA). MTA arose as an antidote to the ‘last click’ attribution model that credited only the final interaction for a sale or conversion (and was also responsible for the disproportionate advertising dollars thrown to Google and Facebook, but that’s another story). With the ability to track customer interactions across multiple digital touchpoints, MTA promised a more nuanced view of the customer journey, assigning value to each touchpoint that contributed to the final conversion. This granularity was a significant step forward, enabling marketers to understand and optimize their digital channels at a micro-level.
However, as digital marketing continued to evolve, the limitations of MTA became more apparent. The model struggled to account for offline channels, long-term effects like brand building, and various external factors impacting customer behavior. The decline of third-party cookies and heightened data privacy regulations also posed challenges, limiting the tracking capabilities that MTA relies upon. These challenges led marketers to reassess MTA’s effectiveness, leading to a shift towards more holistic models such as Unified Marketing Measurement and advanced MMM techniques, which better account for the full range of online and offline marketing influences.
However, for advertisers who are wholly reliant on digital advertising, there is still life in the MTA approach, especially if coupled with RescueMetrics, which fixes the cookie degradation issue easily and effectively.
Traditional MMM
The traditional approach to MMM has drawbacks and limitations. They are usually difficult and time-consuming to update, and they only operate on a channel level, making the insights very high level and infrequent. That was all good and well in times gone by, but this traditional approach to MMM isn’t able to offer the right level of insights needed for the fragmented media market, complex customer journeys, and a range of changing external factors (pandemics, wars, cost of living etc).
This is because traditional MMM is based on linear regression and it models every variable as independent (i.e. you’re modelling a response curve for each publisher, but not accounting for the synergy effects of the mix of publishers and/or channels. This makes it impossible to detect cause and effect and forces a linear interpretation of a very non-linear world. On top of that, these models are dependent on human interpretation, they take time to develop and refit – a factor that became painfully obvious when the impacts of the pandemic-related changes in media consumption, media pricing and consumer behaviour made traditional MMM quickly out of date.
AI-based Marketing Mix Modelling
This complexity of analysing and optimising the effects of fragmented media investments means that the task is no longer analysing response curves and optimising marginal costs. Instead, it is now a game of balancing exponentially high numbers of data points representing the interconnected reality of how media insertions work and contribute to sales, accounting for correlations of each new day of incoming data.
No human is a match for this complexity and multi-dimensionality, which is why AI-based MMM has gained momentum (long before ChatGPT caught the attention of the public). With AI algorithms doing the heavy lifting (creating billions of scenarios to find the most predictive model of interactions), marketing teams have a solid foundation on which to base strategic decisions about how much to invest in media and where to allocate it.
AI-based Marketing Mix Models are able to:
- Make predictions with a minimum 90% accuracy to improve ROI on marketing spend
- Facilitate agile data-driven decisions of media allocations based on frequently-updated models
- Quantify synergies across different media channels
- Model all the individual factors of macro and external data inputs that can impact sales, and decompose the external factors in detail as per media (e.g. not just quantify the impact of macroeconomic factors, but isolate the CPI vs unemployment rate)
- Quantify the value of brand on the baseline, and even the indirect impacts of brand on media response.
- MMM necessarily requires a lot of historical data, typically 2-3 years. This is so the models can have sufficient variability in media delivery and consumer response so to work out how changes in a channel/publisher/tactic have an impact on sales. Many clients find the multi-year historical data challenging to source and organise, and are also concerned about the vastly different media behaviours, media pricing and purchase patterns during the COVID era.
- MMM is based on media spend and doesn’t have a way to understand the underlying audiences associated with each publisher, and how there may be significant differences in media response by audiences. Media spend is a fairly blunt tool for optimising outcomes.
- Creative, like audience, isn’t typically accounted for (although it is sometimes done with a separate model, rather than incorporated into your sales model). Getting granular insights into how particular creative messages perform across different publishers and the audiences consuming the media too much for MMM.
- Geography can play a huge in media outcomes. A brand may have completely different outcomes in VIC vs QLD, but to get this level of nuance in MMM, you may need to have separate models for each state, connected by an overarching or portfolio model. This can quickly get complicated and expensive.
- Time to onboard, time to update (new results in the platform), and time to refit (new publishers/external factors in the platform) can take longer than many marketers would like. Yes, you may be able to get weekly model updates, but then you’re paying for effectively 52 models. Brands will often sacrifice speed for cost – slower updates and fewer refits – but then be frustrated about how long it takes before they can do anything about shifting the needle on a campaign.
Generative Marketing Attribution / People-based Marketing Attribution
Generative Marketing Attribution is a term that has only recently come into existence. It’s a new category of marketing attribution modelling pioneered by Marketing Evolution (M/E).
Ground Control prefers to call it People-based Marketing Attribution. The technology behind the platform is Generative AI and synthetic data (ie anonymised attributes about people that is statistically representative), but the point of the technology is to uncover people’s media and purchase journeys, which is very different to the MMM approach.
M/E’s solution rapidly delivers the breadth of MMM insights alongside the granular depth of MTA using a single model with unparalleled data input flexibility. This generative approach overcomes the flaws of either MMM, MTA, or their combination by applying Nobel Prize-winning research and advanced AI algorithms to allow marketers to understand the impact of their strategies and make better decisions more quickly, saving money and improving results.
The SaaS platform uses data from individual consumers (advertiser results) and the wider population to understand how people make decisions about spending and consumption. AI is used to understand how seeing an advertisement influences a consumer’s decision, and working backwards from each conversion, every media journey is recreated with media attribution for each step. This approach is used to build models for each goal that a marketer wants to achieve, such as increasing website registrations and sales or improving brand reputation.
Marketers can then answer the big questions about the baseline, incrementality, optimal channel mix, long/short balance and create various planning scenarios, but they can also then get deep into the weeds to understand how a multi-channel screens strategy is performing, how geography impacts outcomes, and which message/format combinations work best for each campaign and channel.